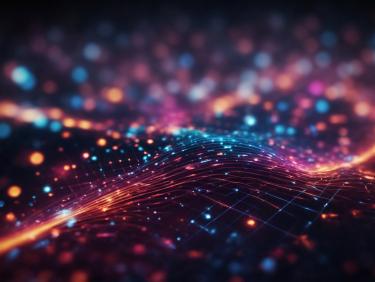
AIPHY
“Challenging AI with Challenges from Physics: How to solve fundamental problems in Physics by AI and vice versa” (AIPHY) ist ein von der Europäischen Kommission im Rahmen der Marie Skłodowska-Curie Actions (MSCA) finanziertes Doktorandennetz. Ziel ist es, optimale Methoden für die KI-gestützte Analyse komplexer physikalischer Daten zu entwickeln, die mit dem Large Hadron Collider am europäischen Forschungszentrum CERN gesammelt wurden.
Aktuelle Themen
Kick-off Meeting für das AIPHY Netzwerk
Das Kick-off Meeting im Oktober 2024 markierte den spannenden Auftakt für das AIPHY Programm. Forschungsteams aus der 4EU+-Allianz kamen in Genf zusammen, um dieses innovative Projekt zu starten. Das Programm basiert auf drei zentralen Säulen: Inverse Probleme, systematische Unsicherheiten und erklärbare Künstliche Intelligenz. Durch die Kombination von Fachwissen im Bereich Künstlicher Intelligenz und Erkenntnissen aus der Teilchenphysik will AIPHY neue Horizonte in der Dateninterpretation eröffnen und neue Wege in der Gewinnung von wissenschaftlichen Erkenntnissen gehen.
Fünf neue länderübergreifende Doktorandennetzwerke an der Universität Heidelberg
Fünf neue länder- und institutionenübergreifende Doktorandennetzwerke an der Universität Heidelberg werden von der Europäischen Kommission im Rahmen der „Marie Sklodowska-Curie Actions“ (MSCA) gefördert. Mit den Möglichkeiten, die die Nutzung Künstlicher Intelligenz zur Beantwortung offener Fragen in der Elementarteilchenphysik eröffnet, befasst sich das Doktorandennetzwerk AIPHY.
Personen
Projekte
Transfer learning for jet energy scales
Include (in-situ) data information into the calibration of jet energy scales (JES) at an early ML-based stage, and improve the jet energy resolution due to considering the correlations among the variables relevant for the JES calibration and to integrating the in-situ constraints at the step of the training of the ML algorithm.
Automated General Searches for New Physics
In contemporary high-energy physics, the number of potential scenarios for physics beyond the Standard Model far exceeds the capacity of dedicated searches. This disparity stresses the need for model-agnostic approaches that explore a broad spectrum of possibilities within the theoretical signal landscape.
General searches with (GNN) AutoEncoders
Develop general Graph Neural Network (GNN) reconstruction algorithm(s) for all event types in experiment; based on this GNN architecture, use Variable AutoEncoder (VAE) to encode the dataset in a latent space (LS); apply the VAE to simulated data, also including theoretically motivated but unobserved physics signatures.
Denoising diffusion probabilistic models
Develop novel methods of inverse problem solutions based on denoising diffusion probabilistic models; investigate new approaches to uncertainty estimation based on stochasticity of denoising diffusion models; introduce new techniques of conditional generation based on concatenation, cross-attention and bias.
New Generative Models for Parton Distributions
The goal of the project is to switch from the current situation, in which a hyperoptimized machine learning model leads to results whose accuracy is tested a posteriori, to interpretable models in which the relation between experimental, theoretical and modeling uncertainty and the uncertainties in the final outcome can be traced using explainable AI tools.
Physics Model-Based AI for Rare Events
Develop a novel strategy to perform reliable extrapolation with machine learning (ML) by space-unwrapping; efficiently handle extreme value modelling via machine learning using model-based strategies like exponentially tiled estimators; solve stochastic partial differential equations via physics informed neural networks for transport equations.
Comprehensive uncertainties for generative models
Develop a method to include uncertainties, starting from Bayesian generative networks; expand strategies to model systematic uncertainties using conditional training on nuisance parameters; extend NNPDF methodology for architecture-driven and parameter-driven uncertainties to generative models; study the effect of guided implicit bias on amplification factors between training and generated sample size.
Explainable AI for Online and Transferable Learning
Develop XAI techniques for online and transfer learning applied to experimental data from physics and signal and image understanding; handle efficiently real-time massive sensor data in online and transfer learning; develop XAI techniques for high robustness and accuracy in multi-sensor environments.
Über uns
Marie Curie Doctoral Networks
Mit den in den „Marie Sklodowska-Curie Actions“ (MSCA) geförderten Netzwerken will die Europäische Kommission zur Ausbildung hochqualifizierter Doktorandinnen und Doktoranden in Europa beitragen. Sie sollen so bestmöglich auf eine Karriere in Wissenschaft und Wirtschaft vorbereitet werden.