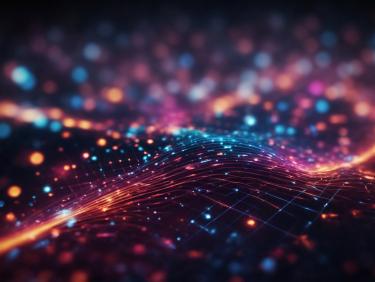
AIPHY
“Challenging AI with Challenges from Physics: How to solve fundamental problems in Physics by AI and vice versa” (AIPHY) is a doctoral network funded by the European Commission in the context of the Marie Skłodowska-Curie Actions (MSCA). The goal is to develop optimum methods for the AI-supported analysis of complex physical data that has been collected with the Large Hadron Collider at the European research center CERN.
Current topics
Kick-off Meeting for the AIPHY Network
October 2024 marked an exciting milestone for the AIPHY program as research teams from across the 4EU+ Alliance gathered in Geneva to kick off this innovative initiative. The program centers around three key pillars: Inverse Problems, Systematic Uncertainties, and Explainable AI. By combining AI expertise and particle physics insights, AIPHY seeks to open new horizons in data interpretation and scientific discovery.
Five New Cross-Border Doctoral Networks at Universität Heidelberg
Five new cross-border and inter-institutional doctoral networks at Heidelberg University are to be funded by the European Commission in the context of the Marie Skłodowska-Curie Actions (MSCA). The potential for using artificial intelligence to answer open questions in elementary particle physics is the topic of the doctoral network "AIPHY".
Persons
Projects
Transfer learning for jet energy scales
Include (in-situ) data information into the calibration of jet energy scales (JES) at an early ML-based stage, and improve the jet energy resolution due to considering the correlations among the variables relevant for the JES calibration and to integrating the in-situ constraints at the step of the training of the ML algorithm.
Automated General Searches for New Physics
In contemporary high-energy physics, the number of potential scenarios for physics beyond the Standard Model far exceeds the capacity of dedicated searches. This disparity stresses the need for model-agnostic approaches that explore a broad spectrum of possibilities within the theoretical signal landscape.
General searches with (GNN) AutoEncoders
Develop general Graph Neural Network (GNN) reconstruction algorithm(s) for all event types in experiment; based on this GNN architecture, use Variable AutoEncoder (VAE) to encode the dataset in a latent space (LS); apply the VAE to simulated data, also including theoretically motivated but unobserved physics signatures.
Denoising diffusion probabilistic models
Develop novel methods of inverse problem solutions based on denoising diffusion probabilistic models; investigate new approaches to uncertainty estimation based on stochasticity of denoising diffusion models; introduce new techniques of conditional generation based on concatenation, cross-attention and bias.
New Generative Models for Parton Distributions
The goal of the project is to switch from the current situation, in which a hyperoptimized machine learning model leads to results whose accuracy is tested a posteriori, to interpretable models in which the relation between experimental, theoretical and modeling uncertainty and the uncertainties in the final outcome can be traced using explainable AI tools.
Physics Model-Based AI for Rare Events
Develop a novel strategy to perform reliable extrapolation with machine learning (ML) by space-unwrapping; efficiently handle extreme value modelling via machine learning using model-based strategies like exponentially tiled estimators; solve stochastic partial differential equations via physics informed neural networks for transport equations.
Comprehensive uncertainties for generative models
Develop a method to include uncertainties, starting from Bayesian generative networks; expand strategies to model systematic uncertainties using conditional training on nuisance parameters; extend NNPDF methodology for architecture-driven and parameter-driven uncertainties to generative models; study the effect of guided implicit bias on amplification factors between training and generated sample size.
Explainable AI for Online and Transferable Learning
Develop XAI techniques for online and transfer learning applied to experimental data from physics and signal and image understanding; handle efficiently real-time massive sensor data in online and transfer learning; develop XAI techniques for high robustness and accuracy in multi-sensor environments.
About us
Marie Curie Doctoral Networks
Through the networks funded in the Marie Skłodowska-Curie Actions (MSCA), the European Commission intends to contribute to training highly qualified doctoral candidates in Europe. This way, they are to receive the best possible preparation for a career in academia and industry.